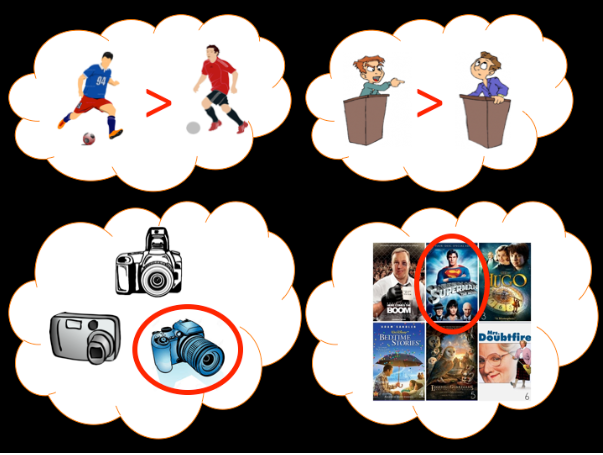
Problems involving learning from ranking and choice data arise in several domains, ranging from sports rankings to elections and from recommender systems to marketing. Indeed, algorithms for these problems have been developed in many different fields, ranging from statistics to computer science and from social choice to marketing. Unfortunately, however, relatively little is currently understood about the learning properties of these algorithms and how they relate to each other.
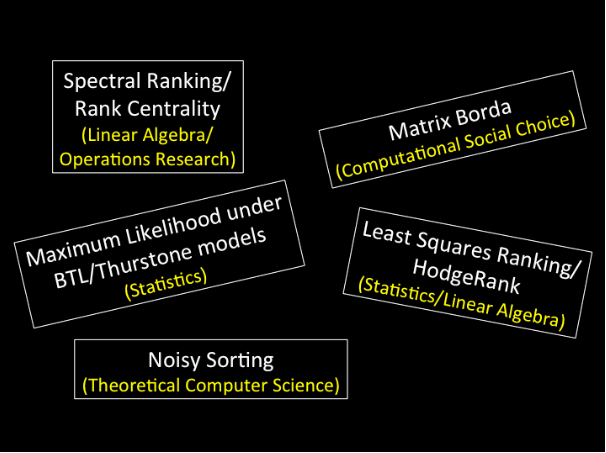
This project develops fundamental theory for ranking and choice modeling that cuts across different fields, and designs new machine learning algorithms for ranking and choice modeling with both strong computational properties and rigorous statistical guarantees.
Faculty
- Shivani Agarwal (CIS)
Students and Postdocs
- Arpit Agarwal
- Nicholas Johnson
- Prathamesh Patil
Funding
- NSF