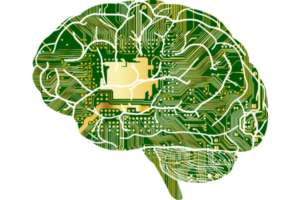
This paper investigates how well people can tell if text they’re reading was written by a machine instead of a human, especially when part of the text is human-written and later transitions to machine-generated. The study reveals that while many people find it hard to detect this switch, some perform better than others, and they can improve over time with practice and motivation.
The researchers also explore factors like model size and text type to see how these impact detection ability. They collected over 21,000 examples where participants pointed out errors, showing that certain types of text make it easier or harder to spot machine-generated content. They also provide a new dataset to help further research in this area.
The study was the subject of an interactive CNN feature in July 2023.
For further information: https://www.cis.upenn.edu/~ccb/publications/real-or-fake-text-analysis.pdf
Faculty
- Chris Callison-Burch
Students and Postdocs
- Liam Dugan
- Daphne Ippolito
Funding
- DARPA KAIROS
- DARPA LwLL Program
- NSF